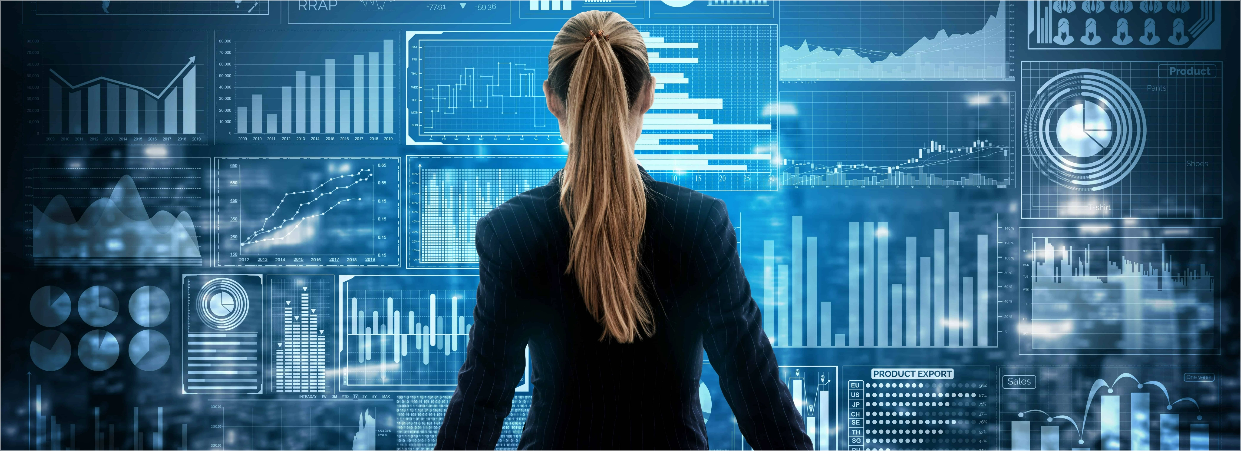
Driving Success with Data: Achieving High Accuracy and Diversity Goals for Clients through AWS Partnership
People Tech collaborated with a prominent client to tackle challenges related to Gender Equity, Recruitment Recommendations, and Increasing Diversity in Recruiting. By leveraging the capabilities of AWS, People Tech implemented robust solutions, incorporating data analysis, exploratory data analysis (EDA), and deep learning algorithms. This case study highlights the problems faced by the client, the solutions implemented using AWS services, and the remarkable outcomes achieved through this partnership.
Problem
The client encountered obstacles in the domains of Gender Equity, Recruitment Recommendations, and Increasing Diversity in Recruiting. These challenges demanded accurate predictions, pattern recognition, and data-driven insights to drive diversity initiatives, recommend suitable job positions and candidates, and achieve high accuracy in key use cases. The existing processes and datasets proved insufficient, necessitating a data-centric solution.
Solution
People Tech, in partnership with AWS, delivered a comprehensive solution to address the client’s challenges:
Gender Equity
- Identified strong signal data features for each use case.
- Conducted exploratory data analysis (EDA) to uncover vital insights and patterns.
- Leveraged diverse datasets, including Census data, LinkedIn, and other open datasets, to enhance prediction accuracy.
- Employed deep learning algorithms, such as RNN (Recurrent Neural Network) and LSTM (Long Short-Term Memory), along with Tensor Flow, for effective model development. est accuracy.
Recruitment Recommendation System
- Identified crucial data elements from HR, Recruitment, and Engineering projects.
- Conducted exploratory data analysis (EDA) to extract valuable insights and patterns from the combined dataset.
- Utilized deep learning and recommendation algorithms to recommend appropriate job positions and candidates.
- Applied deep reinforcement learning techniques to achieve a high confidence level of approximately 91% over time.
Increasing Diversity in Recruiting
- Identified significant signal data features specific to each use case.
- Conducted exploratory data analysis (EDA) to reveal critical insights and patterns.
- Leveraged datasets, including Census data, LinkedIn, and other open datasets, to improve prediction accuracy.
- Utilized deep learning algorithms, such as RNN and LSTM, in conjunction with Tensor Flow, to achieve the highest accuracy.