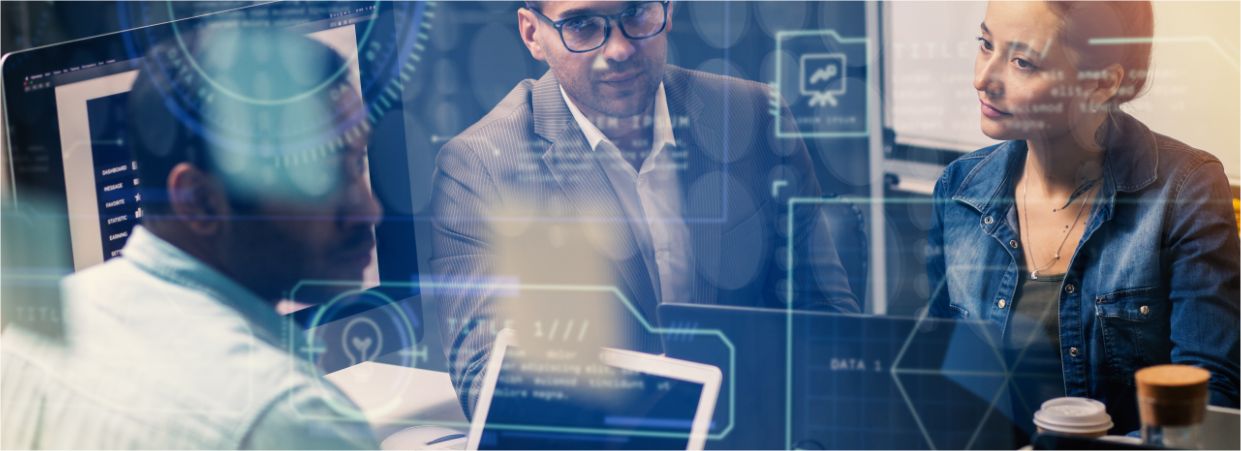
Unsupervised ML for Anomaly Detection: Identifying Unusual Transactions
Introduction
Anomaly Detection using Unsupervised ML is a powerful solution that enables businesses to identify and understand unusual transactions. By providing key features and their weightage for each transaction, this solution allows businesses to pinpoint the specific columns responsible for anomalies and assess their impact. Deployable on Apache Spark, it efficiently processes large datasets in both batch and streaming environments. By categorizing the anomaly’s key columns as high or low, businesses can gain valuable insights into their operations and make data-driven decisions.
Problem
Understanding the reasons behind unusual transactions is crucial for businesses. Existing solutions lack the ability to identify the key features and their weightage that contribute to anomalies, leaving organizations in the dark about the underlying causes.
Solution
Anomaly Detection using Unsupervised ML offers a comprehensive solution with the following capabilities:
Key Column Identification: The solution provides the key column names along with their weightage for each transaction, allowing businesses to determine which columns are responsible for the anomaly and the extent of their impact.
Apache Spark Deployment: Leveraging Apache Spark, the solution seamlessly integrates into data pipelines, enabling efficient processing of large datasets in both batch and streaming scenarios.
Categorical and Numeric Data Support: The solution accommodates both numeric and categorical data, making it versatile and applicable across various domains.
Anomaly Categorization: The solution classifies the key column impact as extremely low, very low, low, normal, high, very high, or extremely high. This categorization provides a clear understanding of the severity of anomalies.
Results
Implementing Anomaly Detection using Unsupervised ML yields the following results:
Unsupervised Machine Learning: The solution does not require training or labeled data, making it highly efficient and eliminating the need for manual data labeling.
Wide Range of Applications: It can be used to identify various insights, such as the best and worst employee performance, most valuable customers, and top-performing products.
Improved Accuracy: Compared to other solutions, Anomaly Detection using Unsupervised ML demonstrates 15% to 20% higher accuracy, ensuring more reliable anomaly detection.
Handling Numeric and Categorical Data: The solution’s versatility allows it to handle both numeric and categorical data, accommodating a wide range of use cases.
Wide Range of Applications: It can be used to identify various insights, such as the best and worst employee performance, most valuable customers, and top-performing products.
Improved Accuracy: Compared to other solutions, Anomaly Detection using Unsupervised ML demonstrates 15% to 20% higher accuracy, ensuring more reliable anomaly detection.
Handling Numeric and Categorical Data: The solution’s versatility allows it to handle both numeric and categorical data, accommodating a wide range of use cases.
Conclusion
Anomaly Detection using Unsupervised ML provides businesses with a powerful tool to identify and understand unusual transactions. By providing key features and their weightage, this solution enables businesses to pinpoint the contributing columns, categorize the severity of anomalies, and make informed decisions. With its accuracy, versatility, and applicability to both numeric and categorical data, Anomaly Detection using Unsupervised ML empowers organizations to uncover valuable insights and enhance their operations.